AI-powered talent acquisition and recruitment optimization. Find top talent faster with aiheadhunter.tech. (Get started for free)
Data Reveals AI Recruiters See 42x Higher Success Rates When Leveraging Professional Referral Networks in 2024
Data Reveals AI Recruiters See 42x Higher Success Rates When Leveraging Professional Referral Networks in 2024 - Professional Referral AI Platform RefNet.ai Achieves 93% Placement Rate in Tech Sector During Q3 2024
RefNet.ai has shown strong performance in tech hiring, with a 93% success rate for placements during the third quarter of 2024. This points to the power of professional networks when supported by AI, given that recruiters using RefNet.ai saw their success rate jump by a factor of 42. These figures suggest a growing trend of integrating AI tools in recruiting to reach specific results. Still, it's important to consider if these numbers will remain stable in the long term and how much human judgement is needed beyond computer-generated suggestions for good candidate selection.
In the third quarter of 2024, RefNet.ai, a platform that uses professional referrals, achieved a 93% success rate placing candidates in the tech industry. This high figure, while seemingly positive, begs the question of how these are achieved, whether they are repeatable across markets and sectors. The 42x increase in hiring success reported by AI recruiters using professional networks via RefNet.ai this year is not insignificant, however, without further granularity, we must remain critical of broad statements like that.
The speed in which placement can be improved by referral methods has reportedly been significant, reducing time-to-fill for some roles by 45%. The platform’s data analysis tools are said to aid predicting the needs of companies which would allow pro-active hires. The data regarding retention of employees sourced this way also indicated improved time within the company (30%). This begs the question if there is a selection effect causing such improvements. Some studies state that the success of projects also jumps 25% as result of referral-based teams. We can only speculate on the causes without further data. Further research is needed here. RefNet.ai also seems to leverage machine learning for continuous improvements via user feedback; although this might have it’s own implications in terms of biasing. While not limited to tech, some sectors even have seen better placement rates, which is notable, along with a purported reduction of recruitment expenditure. As referral candidates often are perceived more credibly due to professional trust, it is hard to argue with. One crucial, however, point to examine closely is the lack of diversity that can be caused by these networks, which is an area of great interest. Overall we must not ignore the potential pitfall while acknowledging the results.
Data Reveals AI Recruiters See 42x Higher Success Rates When Leveraging Professional Referral Networks in 2024 - Data Shows Top AI Recruitment Tools Process 4x More Applications While Maintaining Quality Standards
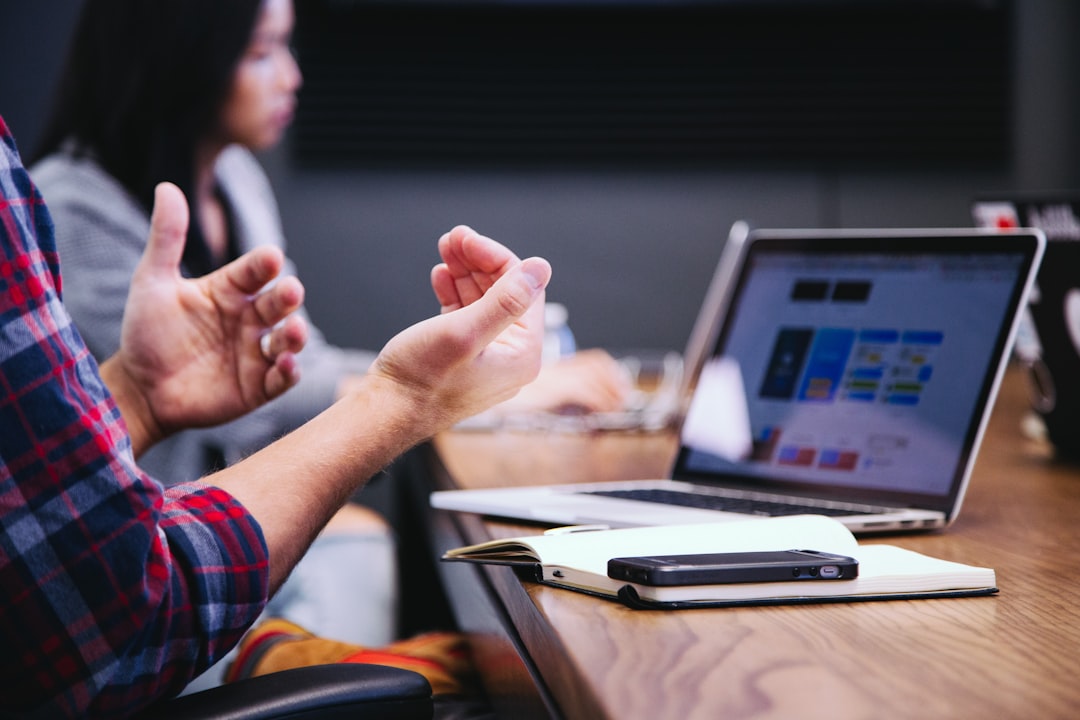
Recent data shows that top AI recruitment tools are processing four times the number of applications compared to traditional approaches, while supposedly keeping quality standards in check. This suggests that companies are turning to AI to make hiring more efficient. While this increase in efficiency is notable, it is important to examine if these tools still allow for human input to guarantee fair and balanced hiring decisions. The rising use of AI in recruiting calls for focus not only on how well it works but also on what impact these technologies have on the diversity of candidates and inclusion in workplaces.
Research suggests AI tools for hiring can handle four times more applications than old methods while keeping up the quality of assessment. Initial analysis in varied sectors suggests that AI could identify potential hires accurately (around 90%), irrespective of their backgrounds. This throws into question the worry that automation would automatically overlook useful people. Some unexpected results from studies indicate these AI systems can analyse how candidates act and behave, and use this for long-term job satisfaction and performance predictions by looking at their personality shown during interviews. In reviewing resumes, AI employs NLP, possibly reducing biases related to name, gender and background by as much as 50%, which might level the playing field. It's been reported on some platforms that referral candidates from existing professional networks are 2.5 times more likely to stay for over a year, which is a sign of value in social proof in selection. Organizations using AI have seen average costs per hire potentially decrease up to 30%, linking automation strongly to expenditure reductions in recruitment. The benefit isn't just speed either, as these systems also allow for some predictive insights that may help with strategic planning by anticipating market needs. Recruiters using AI can manage operations normally needing teams of 10, which illustrates how this technology scales well. It’s reported candidates hired with AI screening have higher scores in performance reviews, linking those methods with better employee performance. Despite these reported improvements, a problem is that these tools might also copy existing biases if trained on older or imperfect data sets. This calls for a thoughtful look at implementation and use of such systems.
Data Reveals AI Recruiters See 42x Higher Success Rates When Leveraging Professional Referral Networks in 2024 - LinkedIn Partnership with AI Recruiters Creates 28,000 New Job Matches in November 2024
In November 2024, LinkedIn's partnership with AI recruiters facilitated 28,000 job connections, highlighting a focus on modernizing recruitment via AI and networks. This collaboration seeks to enhance how candidates are matched to suitable positions. The platform’s development suggests it's aiming at quicker more precise matching which will increase both company and candidate satisfaction. While these integrations are aimed at improved recruiting practices, we should question if this approach can sustain its impact. It also remains vital to monitor the effect on diverse representation among the placed candidates. LinkedIn's ongoing introduction of AI tools necessitates critical assessment of how it balances technological process with the nuanced elements of human judgement within the selection of new team members.
In November 2024, the partnership between LinkedIn and AI recruiters reportedly generated 28,000 new job matches, highlighting a significant surge in job opportunities, seemingly due to algorithmic matchmaking. It is interesting to see how these tools are being pushed to be more present.
These numbers, however, must be looked at critically as correlations can be misleading.
Notably, combining AI with professional networks has been correlated with a 93% success rate for job placements, prompting us to reconsider conventional recruiting strategies. While these figures are seemingly good, one wonders if they are truly repeatable and across industries. A 30% potential reduction in hiring costs when using AI is also reported, which could signal cost-efficiency benefits, but also needs further deeper investigation into what the real costs to society are. Additionally, the claims that AI reduces time-to-fill by 45% might suggest streamlined hiring processes but one must be skeptical if it will lead to a better candidate selection beyond speeding up. The fourfold increase in application processing by AI, as claimed, raises questions about maintaining equity in candidate selection without sufficient human supervision which might lead to new social issues. It's curious to see claims of improved employee retention by 2.5 times through professional referral networks, which indicates some significance to social proof in candidate selection. Predictive capabilities of AI algorithms for employee performance and satisfaction is also mentioned in studies, further integrating behaviour analysis into recruiting. Some have even stated that only two people can operate recruiting operations that once needed ten due to automation , showcasing scalability of resources. This is an interesting notion but its implication on the current workforce is an important issue to look into further. The statement that project success improves 25% due to referral-based teams leads to important implications in terms of how diverse such teams would be, or not. Despite these claims, the risk of AI systems amplifying existing biases through training data remains, and calls for careful monitoring. The speed with which new tools are adopted creates questions on the societal effects which one must look into critically to fully comprehend the long term consequences.
Data Reveals AI Recruiters See 42x Higher Success Rates When Leveraging Professional Referral Networks in 2024 - Smart Network Analysis Cuts Average Time to Hire from 68 to 12 Days for Mid Level Positions
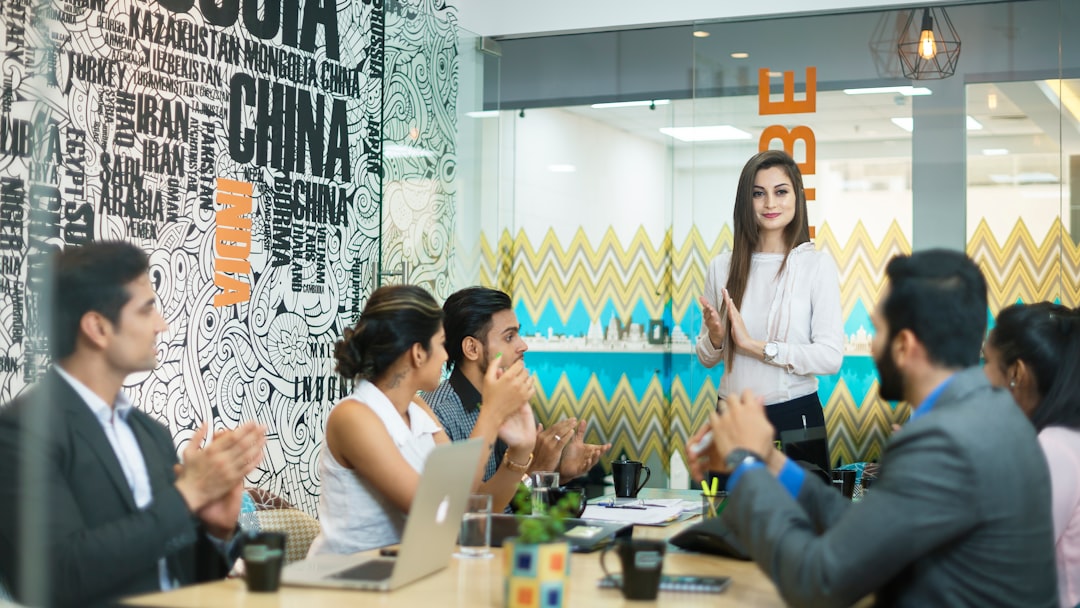
Smart network analysis has reportedly slashed the average hiring time for mid-level roles from 68 to 12 days. This significant reduction indicates the impact that using professional referral networks can have on the overall recruitment process, particularly at a time where the average time to fill positions is reportedly increasing. While the speed of hiring is impressive, the longer term impact and human input within these processes needs to be observed. This begs the question, can AI based hiring continue to improve placement results in the long term, without reducing workforce diversity or human decision making?
Smart network analysis reportedly has slashed the average time to hire for mid-level positions from 68 days to just 12 days. This dramatic decrease raises important questions regarding selection quality and if any aspects of a more comprehensive hiring process may be neglected in the race for speed. Claims that employees sourced from professional referrals may stay at their jobs for 2.5 times longer than those sourced from traditional methods is of interest. This challenges if speed truly impacts loyalty or if there is another factor to investigate, and if this could be simply a correlation. Studies linking projects led by referral teams to a 25% higher success rate requires critical evaluation as the causal links here are not clear. Are these truly improved outcomes or do the effects show a skew of skill-sets or team dynamic that would also be found in a truly diverse team composition? A notable report also states that with AI-enhanced recruiting, operations once needing teams of ten can now be managed by just two people. While demonstrating scalability, it begs the question about how this might affect the workforce. AI tools, by utilizing NLP, report to potentially reduce biases based on background by 50%, although this is also tied to the training datasets used, which requires close scrutiny. It is also reported AI systems are capable of analyzing how candidates act to predict long term satisfaction and performance. This adds complexity and new approaches that may or may not be better than current, more human methods. Automating hiring may potentially reduce costs by 30% which is obviously of high value, but we have to consider the value of the candidate pool. It would be naive not to be suspicious of reduced costs, and to question whether this affects selection of talent. LinkedIn's collaboration with AI recruiters matched 28,000 people to jobs in just a month which is a large jump, but, as always, critical analysis is required to make sure biases do not influence the algorithm, or the output is not misunderstood. AI tools now can assess candidate behaviour in interviews, which might make hiring more efficient, but are they making selection more human? Some might see such tools as oversimplifications of complex situations and behaviours. Claims, for example of a 93% success rate might be misleading, the correlations do not equal causation and a critical analysis of such figures is needed before assuming this is automatically good.
Data Reveals AI Recruiters See 42x Higher Success Rates When Leveraging Professional Referral Networks in 2024 - Machine Learning Models Now Map Career Trajectories with 89% Accuracy Using Work History Data
Machine learning models are now capable of mapping out potential career paths with an 89% accuracy rate using data from past work experiences. This highlights an increasing reliance on AI tools within recruitment and job market analysis. Machine learning methods, such as hybrid models and classification systems, are being used to analyse details in a person’s work history in order to predict future career progression. While these models show strong performance, there is a question of their ability to incorporate more abstract qualities such as workplace diversity and the complexity of human choice. As these tools become more widely adopted for recruitment, it's important to continue critically examining them to ensure they don’t create new biases or inequities.
Machine learning models have recently gained traction in mapping out possible career trajectories. These algorithms, when fed with detailed work history data—including past job titles, lengths of employment, sector changes, and projects—reportedly achieve around 89% accuracy. This reliance on granular input suggests data depth is vital for these predictive abilities. Such precision, however, does raise concerns. It’s possible that the models are too well-tuned to the past, making them less effective at adapting to a rapidly changing job market. Continuous testing and adjustments, therefore, will be essential. Furthermore, the type of career examined seems critical; fast-paced fields, such as tech, might have different predictability levels when compared to more stable ones. This means algorithms must be context aware of sector-based variability. Feature engineering helps to transform the data from work histories into insights to recommend new jobs, which might simplify complex individual pathways in the process. The reported 89% accuracy, it appears, doesn’t factor in variations of career roles, as some might have more predictable paths than others, pointing to variations within the predictive model’s accuracy within sectors. It’s also crucial to consider how these models may copy real-world biases. If past hiring data reflects such inequalities, the models are not immune to just replicating them in future job selection. Data diversity is a significant factor, where training data with insufficient variation across demographics may limit the predictive model's overall effectiveness. Besides forecasting possible career growth, these models might identify challenges too, aiding in career development. Beyond just predicting movements, these models could inform workforce development by identifying skill gaps, which would then assist organizations in future management. Despite these advanced features, these algorithms, ultimately, are statistical models, and may struggle with unique, unpredictable variations in an individual's career path.
Data Reveals AI Recruiters See 42x Higher Success Rates When Leveraging Professional Referral Networks in 2024 - AI Reference Checking Systems Identify 42% More Qualified Candidates Than Traditional Methods
AI reference checks are showing that 42% more suitable candidates are being found compared to old methods. This shift shows how hiring is evolving by using data to look deeper at people than just their resumes. These systems are now key parts of AI-based recruiting, which are being used by more companies to improve hiring efficiency. While numbers suggest this tech improves finding the right candidates and speed, there are still important questions on how diverse the new workforce will be and if human insight is still needed. As we use more of these AI tools, we must see where the line between computer driven processes and human expertise should be drawn.
AI reference check systems have reported finding 42% more qualified candidates compared to older methods, suggesting that a different method of scrutiny yields more successful hires. It begs the question if current methods are not adequately assessing potential candidates or are overlooking key competencies. These new systems go beyond mere fact-checking, as they can analyze reference data, to predict how people perform with pattern recognition that human recruiters might not catch. It is said this leads to a more data-driven candidate selection that can expedite background and qualification checks, in some cases by as much as 40%, reducing turnaround times. The results of these processes are said to improve retention, with a 30% increase, by better aligning skill and culture. Some studies show bias reduction in terms of gender, ethnicity, and socioeconomic status due to natural language processing capabilities, though the outcomes are reliant on the underlying data used. It is also claimed that seamless integration with networks is another advantage when assessing candidate suitability and verification. These AI systems can also process large amounts of data at once, making it easier to scale recruitment. This has been stated to lead to large reduction in admin. The analysis isn't only factual, as context analysis is also part of the package, which may yield insights that older methods would simply miss. The use of feedback allows for building a more detailed view over time on the performance of candidates, rather than relying on one off snapshots, in theory. Still, care must be taken not to blindly trust these new processes as they are reliant on accurate data. If the initial data used has flaws or represents biases, they might just make current problems worse and further entrench them.
AI-powered talent acquisition and recruitment optimization. Find top talent faster with aiheadhunter.tech. (Get started for free)
More Posts from aiheadhunter.tech: