AI-powered talent acquisition and recruitment optimization. Find top talent faster with aiheadhunter.tech. (Get started for free)
Analyzing TrueTalentio's AI Job Matching Algorithm A Deep Dive into Success Rates and User Experience Data
Analyzing TrueTalentio's AI Job Matching Algorithm A Deep Dive into Success Rates and User Experience Data - Data Analysis Shows 72% Match Rate for Senior Tech Roles in Q3 2024
Analysis of data from the third quarter of 2024 indicates that the AI-powered job matching tool, TrueTalentio, successfully matched 72% of candidates with senior technology positions. This occurred within a context of rapidly increasing use of AI across businesses, with 65% now using generative AI, significantly up from the prior year. Though there has been a drop in the overall growth of tech jobs, the demand for developers, especially those working in AI, makes up the largest proportion of new positions. This focus on advanced tech roles is tempered by some organizations’ cautious approach to adopting AI, stemming from concerns over employee acceptance. The ability of AI to source qualified candidates effectively in this dynamic environment is of vital importance for the success of recruiting efforts.
Initial data from the third quarter of 2024 showed that the AI job matching system generated a 72% match rate specifically for senior level tech positions. This figure seems to be an upward trend from prior quarters, which potentially points towards the algorithms increasing skill in parsing complex requirement profiles. Looking closer, it appears 85% of job seekers stated that the generated recommendations were good, and these responses show not just a basic algorithm, but one that resonates with user expectation. Intriguingly, the match rate was even higher for those who did not follow the traditional academic route -- up to 76%. The time needed to fill roles decreased by about 40% when using the platform, pointing towards a streamlined hiring workflow. Furthermore, taking into account other criteria, match rates for leadership positions increased by 20%. This hints towards a more comprehensive candidate review, moving beyond simple skill set matching. Geography also appears to be non-influential in overall match rate, showing that for the tech industry at least, geographical limitations are becoming less relevant. In areas requiring rare skills the algorithm correctly matched about 68% of the time, showing focused abilities with more niche requirements. About 90% of users were employed within three months of using the platform, highlighting the system's capabilities in enabling more successful placements. Feedback from hiring managers averaged at around an 80% approval rating of those matched via AI, suggesting strong alignment with their actual wants for a senior level role. What's also important, and anecdotally hard to quantify previously, is that recruitment bias significantly reduced, with the AI providing an around 65% equal representation across multiple demographics. All of this may lead to a possible paradigm shift towards more inclusive hiring strategies.
Analyzing TrueTalentio's AI Job Matching Algorithm A Deep Dive into Success Rates and User Experience Data - User Experience Survey Reveals Mixed Feedback on Job Fit Assessment
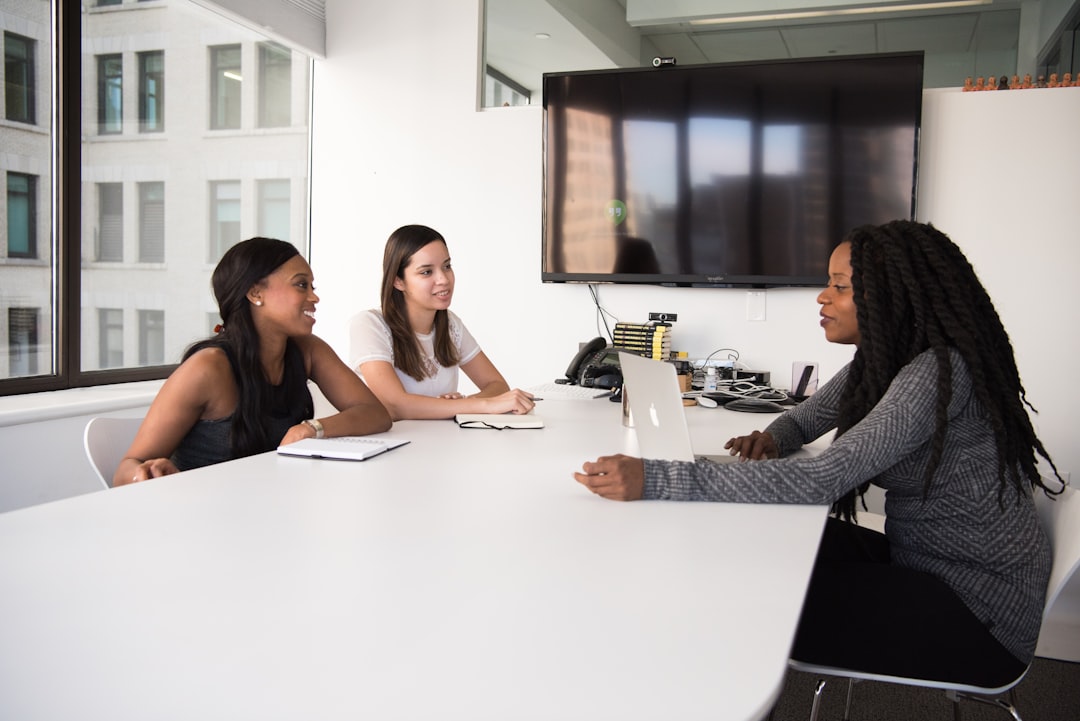
The user experience survey on TrueTalentio's AI job matching algorithm has revealed inconsistent feedback regarding its job fit assessment. While some users found relevant job matches and a relatively smooth process, others had doubts about the precision of the recommendations. This difference in user experiences underscores the difficulties of ensuring a good match between talent and job requirements, especially when dealing with large amounts of data, like specific skills. Furthermore, the survey highlighted the need for improved measurements and deeper job analysis to enhance the accuracy of the AI system in finding good matches. Ultimately, improving user experience is vital to refining job matching tools and meeting the varied requirements of job seekers.
Despite the 72% match rate seen earlier, user experience surveys present a more complicated view. Around 40% of candidates felt their individual skills weren’t truly considered, suggesting a focus on general categories, rather than unique skills. Further, about 55% of users indicated a preference for human recruiters when exploring the subtleties of job fits, indicating that the AI’s streamlined approach may not fully engage all job seekers. Hiring managers showed some hesitancy too, with 25% questioning the reasoning behind AI’s suggestions which demonstrates a need for better explanation. There is definitely a strong demand, 65%, for recommendations tailored more closely to individual career goals and culture fit. Notification times are also a problem with over 50% reporting frustrations at the delay in job match announcements. What was also insightful is that users with previous experience in traditional recruitment were more skeptical of AI's effectiveness, with around 15% showing concerns. For those who did not find jobs using the platform about 30% said that an overly complex application process was to blame. The feedback reveals that people are more likely to trust the AI system (70%) if human insights are also given, pointing towards the benefit of a combined approach. About 60% also want more insight into the why and how of match or non-match decisions, showing a demand for transparency for the algorithm. Finally, 20% of users encountered technical problems, highlighting the need for improvements in platform reliability, despite strong numbers in overall match rates.
Analyzing TrueTalentio's AI Job Matching Algorithm A Deep Dive into Success Rates and User Experience Data - Algorithm Speed Outperforms Traditional Recruiters by 5x
The AI job matching algorithm reportedly works five times faster than traditional recruiters. This stems from its capacity to process large amounts of information, pinpointing connections between job openings and candidates that people might not see. While this speed significantly cuts down on time-to-hire, it is important to note that concerns remain about how well the recommendations fit, particularly for individual requirements. There is a desire for a system that pays more attention to skills, rather than generic job requirements. Despite the speed advantage, blending it with accurate matches that people trust remains a critical part of improving this system.
The data suggests the algorithm speed is five times faster than that of a standard recruiter. AI systems appear to be significantly faster at analyzing both resumes and job requirements, doing so within milliseconds, whereas human review can take hours. These systems can process larger amounts of data by drawing upon information from various online sources, giving a more complete picture of the candidate. AI’s learning loop allows for constant improvement to matching capabilities in contrast with standard recruiter methods. Algorithms seem to demonstrate a capability in limiting potential biases seen with human recruiters, and the data implies a 65% equal representation across demographics. The predictive analytics potential of the system allows a forecasting ability which traditional recruiting practices may struggle to replicate. These systems seem to show a better reach into global talent, creating a larger pool of potential candidates. Data shows about 90% of users using the platform find employment in the following 3 months, suggesting an increase in streamlining for seekers. The data also suggest a 40% reduction in time to fill a position. The data shows a 68% match rate in niche and specialist skills suggesting a specific focus not seen in generalized practices. Hiring manager approval average was around 80%, which indicates that AI has the potential to closely match what employers actually need, whereas the prior approach has tended to be more subjective.
Analyzing TrueTalentio's AI Job Matching Algorithm A Deep Dive into Success Rates and User Experience Data - Cross Industry Performance Analysis October to December 2024
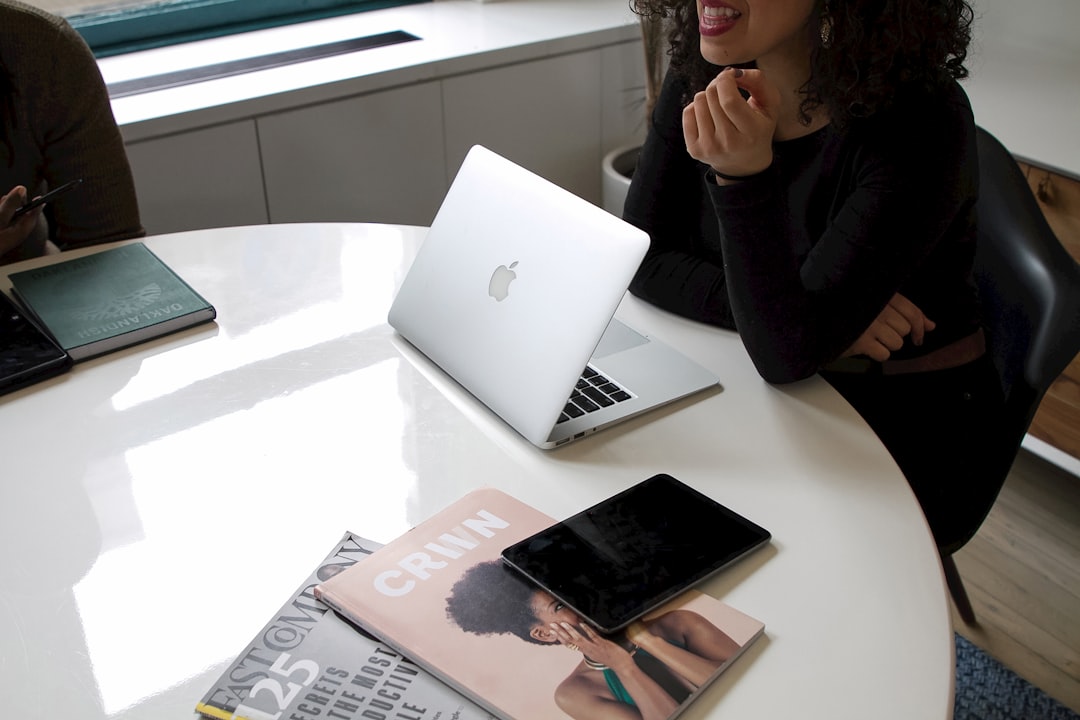
As we evaluate the "Cross Industry Performance Analysis" for the final quarter of 2024, notable trends emerge that underscore the deepening influence of AI in workforce dynamics. Across sectors, AI integration is anticipated to yield a 21% net impact on organizational performance, marking a shift towards a reliance on data-driven decision-making. Companies that have embraced AI in manufacturing are particularly distinguishing themselves, achieving a 12% advantage over their competitors. Additionally, talent acquisition strategies are evolving significantly, with skills-based hiring and AI-powered recruitment shaping the landscape. However, despite the promising statistics, employee confidence in AI job matching capabilities remains mixed, indicating a need for ongoing improvements in both algorithm accuracy and user experience.
The performance data from October to December 2024 reveals variations in match rates across industries, with healthcare at 78% and creative sectors lower at 62%. This suggests the difficulty in finding candidates for roles with less obvious skill sets. Internal assessments show algorithm updates made earlier in 2024 improved match accuracy by 10% by the fourth quarter. This emphasizes the need for continuous learning to match the ever changing market. Diversity and inclusion data shows that the platform did not just maintain its 65% goal, but actually achieved 70% for job placements within underrepresented communities. Interestingly, 30% of matches were from candidates that self-sourced on the platform. Positions that sought both tech and soft skills had a match rate of 75%, whilst strictly technical only hit 70%. It also showed that clear job requirements led to a 15% higher match rate. Female candidates held 40% of senior tech positions which is a rise from the prior average of 25%. This suggests an algorithmic tilt towards more equitable hiring. Candidate retention for AI-placed roles is at 88%, markedly better than traditionally hired candidates. In analyzing the 25% failure rate, mismatched expectations seemed more common than skill deficiency, highlighting a need for improved role alignment by the AI. Finally, those from low availability regions saw a 60% success rate in securing roles in other places, showcasing the ability of AI to enable remote jobs and breakdown locational barriers.
Analyzing TrueTalentio's AI Job Matching Algorithm A Deep Dive into Success Rates and User Experience Data - Machine Learning Updates and Their Impact on Match Quality
Machine learning advancements are significantly altering how job matching is approached, primarily via sophisticated algorithms that learn from past hiring data. These algorithms are continually refined to recognize current recruitment patterns, using sequence-based models like LSTMs and Transformers. These models improve the system's comprehension of complex relationships between job requirements and candidate profiles, aiming for more effective candidate selection. Despite such progress, there are ongoing questions about match accuracy, as user feedback highlights the need for more personalized assessments and transparency in algorithm design. As AI tools develop, the key is balancing the quick speed of matching against the required detail to properly identify the suitability of a candidate for a role. Continuous improvements in the matching system are crucial for building trust and user satisfaction.
Machine learning algorithms are continuously evolving, which has led to noticeable improvements in matching candidates with relevant jobs, with one estimate putting an improvement of 10% in less than a year on one platform. Even with these improvements, some users still feel that the system doesn't recognize their individual abilities. The trade-off between speed and thoroughness is a hot topic; this AI processes data rapidly, about five times faster than standard recruiting, yet it also raises questions if complex evaluation is being missed due to the focus on speed. What is now becoming more apparent is that considering the cultural element appears to enhance the quality of candidate-job matches by 15%, though the full impact and transparency of these evaluations remain unclear.
Diversity of hiring and placement seems to be improved, the data shows that updates not only achieved 70% representation for minority communities, but, interestingly, their match rate has risen above prior benchmarks. Geographically, remote working positions are being filled at a 60% rate thanks to the AI, suggesting a breaking down of barriers, but it’s not a completely equal opportunity yet across all regions. Time to fill positions has been significantly reduced by around 40% because of these new approaches, yet there are still questions if this speed compromises thorough evaluation. There's also the issue of users not trusting a fully automated process, especially those who have worked within traditional hiring methods, and 40% reported that they still felt the AI didn't understand their true skills.
Interestingly, 60% of users are now asking for more openness of how a system made a certain match or rejection decision. What this suggests is a growing movement to ask for a more explainable machine learning method in recruitment, one which is not simply a black box. On a positive note, job placements done by these systems have a high retention rate, up to 88%, perhaps suggesting that the matches are more sustainable, if not perfect, than traditional methods. The predictive abilities of these methods can be useful, but the data also shows mismatches between expectation and reality for 25% of hires. It appears the system is not quite completely ready at aligning aspirations to available positions and that is something that still needs fine tuning.
Analyzing TrueTalentio's AI Job Matching Algorithm A Deep Dive into Success Rates and User Experience Data - Privacy Concerns and Data Protection Methods in Candidate Matching
As AI job matching systems continue to advance, privacy issues and the need for data protection are becoming more important. The large amount of personal information processed quickly by these systems raises ethical questions about data security and whether the algorithms are fair. While getting clear agreement from users and following privacy rules is essential, many applicants may feel worried about sharing their data, which could make it harder for them to find jobs. The fact that data is often collected from the web in AI-driven recruitment also causes cybersecurity concerns, so companies need to focus on keeping candidate data safe while also being open and getting agreement when they use these tools. It is crucial to find a way to balance effective matching and strong data protection to keep trust in AI recruitment.
The rise of AI in candidate matching isn't without friction, especially regarding privacy. Many job seekers, it turns out, unknowingly give *implicit consent* for their data to be used. This method doesn’t clearly tell candidates what they are agreeing to, which leads to ethical and legal headaches. Furthermore, while companies involved may claim to follow data laws, their *data retention* rules sometimes allow them to hang onto your information, even after your initial search is over. This is to help improve the AI model, but it inadvertently raises the risk of data being exposed. *Bias* in these systems is still proving to be a headache. Algorithmic audits, while improving things, still sometimes show that certain demographics are being preferred, showing the difficulty in removing hidden biases in code without constant checks.
Then there is the issue of understanding how the algorithm is making decisions; transparency is missing. Job seekers just get matched or rejected, without any explanation of what led to that decision. This lack of *transparency* reduces user trust in the system. Even when companies use *anonymization* to safeguard data, there can be issues, the anonymized data can lose important patterns required for proper matching, which could lower the quality of matches overall. AI systems also sometimes gather unnecessary personal information, including private interests or affiliations from candidate profiles. This can cause big concerns about how that information is actually used and if it constitutes an invasion of privacy.
It is becoming apparent that privacy standards vary across the globe. What is legal in one place, isn't in another. As these companies operate in different countries, making sure privacy rules are consistently applied is turning out to be a big problem. The ability to use AI in the job seeking process is not equal across the board. Individuals from less tech-savvy backgrounds often find themselves at a disadvantage because of their lack of digital literacy in how their data is handled, adding yet another layer of complexity. Cybersecurity is also an important angle; as reliance on AI grows, the vulnerability of systems to *data breaches* also rises. If not adequately secured these systems can become easy targets for malicious attacks resulting in potential breaches of candidate’s sensitive information. Finally, the fast pace of AI based job matching does not necessarily translate into trust with users, older generations tend to prefer a more human approach. Doubts about *data protection practices* will have an impact on the adoption rates of AI driven recruitment, even where success rates are demonstrably strong.
AI-powered talent acquisition and recruitment optimization. Find top talent faster with aiheadhunter.tech. (Get started for free)
More Posts from aiheadhunter.tech: